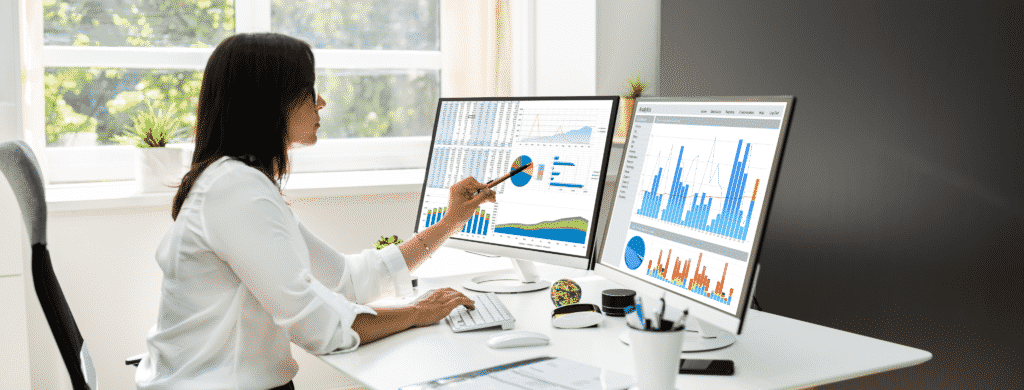
In the active technological landscape of 2025, entry-level data analysis emerges as a gateway to transformative career opportunities, offering aspiring professionals a profound pathway into the world of strategic decision-making and technological innovation. As organizations increasingly rely on data-driven insights, entry-level data analysts become crucial architects of organizational intelligence, bridging raw information with strategic business capabilities.
Professional Landscape of Entry-Level Data Analysis
Entry-level data analysis represents a sophisticated technological discipline that integrates foundational computational skills, statistical methodologies, and strategic problem-solving capabilities. Professionals in this field leverage emerging digital technologies to transform complex datasets into actionable insights, creating value across diverse organizational ecosystems while developing critical analytical capabilities.
The contemporary data analysis ecosystem represents a dynamic and rapidly evolving professional domain, characterized by unprecedented growth and technological transformation. Organizations across diverse sectors—including technology, finance, healthcare, retail, and digital marketing—are increasingly recognizing the strategic value of data-driven decision-making. Entry-level data analysts are now pivotal contributors, bridging the gap between raw data and strategic insights, utilizing sophisticated analytical methodologies, advanced computational tools, and robust statistical frameworks to extract meaningful patterns and actionable intelligence from complex datasets.
Key Concepts to Learn
Advanced foundational methodologies encapsulate the core framework of entry-level data analysis, providing emerging professionals with sophisticated techniques to decode complex datasets. These methodologies extend beyond basic statistical approaches, integrating fundamental machine learning concepts, introductory predictive modeling, and computational techniques. By mastering these initial analytical frameworks, entry-level data analysts can generate meaningful insights that support strategic organizational decision-making.
Foundational Statistical Methodologies: Master introductory statistical techniques, including descriptive statistics, basic regression analysis, and fundamental data interpretation strategies.
Entry-Level Data Visualization Technologies: Develop initial expertise in visualization tools like Tableau Public, Google Data Studio, and basic Excel visualization capabilities.
Introductory Predictive Modeling Frameworks: Learn fundamental machine learning algorithms and basic statistical inference techniques suitable for junior analysts.
Digital Collaboration Technologies: Gain a comprehensive understanding of entry-level data processing tools, including spreadsheet technologies and introductory cloud-based platforms.
Strategic Data Analysis Integration: Develop foundational skills in transforming basic analytical insights into preliminary organizational recommendations.
Educational Pathway
The academic trajectory for aspiring data analysts has become increasingly sophisticated and multidisciplinary, encompassing comprehensive undergraduate and graduate programs specifically designed to cultivate advanced analytical competencies. Traditional academic disciplines like statistics, mathematics, computer science, and information systems have evolved to incorporate specialized data science and analytics curricula. These programs strategically integrate theoretical knowledge with practical skill development, offering students extensive exposure to programming languages, statistical modeling, machine learning algorithms, data visualization techniques, and critical analytical methodologies. Additionally, many institutions now collaborate directly with industry partners to provide internship opportunities, capstone projects, and real-world problem-solving experiences that prepare students for the complex demands of modern data analysis roles.
Specialized Learning Programs: Pursue associate degrees or certificate programs combining statistics, computer science, and entry-level business intelligence curricula.
Professional Foundational Certifications: Obtain entry-level credentials from platforms like Google Data Analytics Professional Certificate and Microsoft Excel Data Analyst Associate.
Online Learning Ecosystems: Leverage accessible platforms like Coursera, edX, and specialized digital learning providers.
Practical Research-Oriented Training: Engage in introductory research programs and digital initiatives providing hands-on analytical experiences.
Continuous Learning Infrastructure: Develop proactive approaches to professional development through online workshops and webinars.
Practical Experience
Developing practical experience has become a critical differentiator for entry-level data analysts seeking to establish themselves in a competitive professional landscape. Beyond traditional academic projects, aspiring professionals are increasingly leveraging diverse platforms and opportunities to demonstrate their analytical prowess. Online learning platforms like Coursera, edX, and DataCamp offer comprehensive certification programs, while competitive platforms such as Kaggle provide opportunities to participate in data science challenges and collaborative projects. Personal portfolio development through GitHub repositories, participation in open-source data analysis projects, and engagement with professional networking platforms like LinkedIn have become essential strategies for showcasing technical skills, demonstrating problem-solving capabilities, and building credibility within the data analysis community.
Personal Analytics Projects: Develop introductory data analysis projects using publicly accessible datasets.
Kloudhire Platform: Leverage innovative platforms connecting emerging data analysis professionals with initial project opportunities.
Open-Source Contributions: Participate in entry-level data science community projects.
Initial Research Collaborations: Seek opportunities with local organizations focusing on foundational analytical methodologies.
Digital Learning Platforms: Engage with specialized platforms providing practical problem-solving experiences.
Skills to Develop
The skill set required for contemporary entry-level data analysts has become increasingly sophisticated and multifaceted. Technical proficiencies now extend beyond traditional programming languages like Python and R to include advanced statistical analysis, machine learning techniques, and comprehensive data manipulation skills. Proficiency in database management systems, particularly SQL and NoSQL databases, is crucial. Data visualization tools such as Tableau, PowerBI, and advanced Excel functionalities are now standard expectations. Equally important are advanced analytical competencies including predictive modeling, statistical inference, experimental design, and sophisticated data cleaning and preprocessing techniques. Critically, successful data analysts must also cultivate robust soft skills, including complex problem-solving, strategic communication, cross-functional collaboration, and the ability to translate intricate technical insights into compelling narratives for diverse stakeholders
Foundational Programming Proficiency: Master entry-level programming languages like Python and SQL with basic data manipulation skills.
Introductory Analytical Techniques: Develop a comprehensive understanding of fundamental statistical analysis strategies.
Digital Collaboration Technologies: Gain initial expertise in collaborative data processing platforms.
Strategic Communication Skills: Cultivate abilities to present basic analytical insights effectively.
Foundational Data Storytelling: Develop capabilities to communicate initial data-driven observations.
Job Opportunities in Entry-Level Data Analysis
The job market for entry-level data analysts has expanded exponentially, presenting a diverse and dynamic landscape of professional opportunities. Traditional roles such as Data Analyst, Business Intelligence Analyst, and Research Analyst have been complemented by emerging specialized positions in sectors like digital marketing, healthcare technology, financial technology, and e-commerce. Emerging domains such as artificial intelligence, machine learning, Internet of Things (IoT), and predictive analytics are creating novel career pathways. Compensation structures reflect the high demand for analytical talent, with entry-level salaries typically ranging from $50,000 to $75,000, and potentially escalating to $90,000 in technology hubs and specialized industries. Moreover, the global shift towards data-driven decision-making ensures continuous growth and evolution of career opportunities in this exciting professional domain
Junior Data Analyst: Perform initial data collection and basic analytical tasks.
Business Intelligence Associate: Support organizational reporting and preliminary insight generation.
Data Reporting Specialist: Create foundational reports and dashboards.
Entry-Level Research Analyst: Conduct initial data investigations and support research initiatives.
Junior Digital Insights Coordinator: Support strategic decision-making through basic data analysis.
Conclusion
The journey to becoming an entry-level data analyst in 2025 represents a transformative expedition of continuous learning and technological exploration. By developing a strategic approach to education, gaining practical experiences, and cultivating an adaptable skill set, professionals can position themselves at the forefront of a revolutionary technological workforce.
As digital technologies continue to reshape organizational landscapes, entry-level data analysts will play a critical role in driving initial strategic innovations and generating foundational insights across diverse professional domains.
0
0