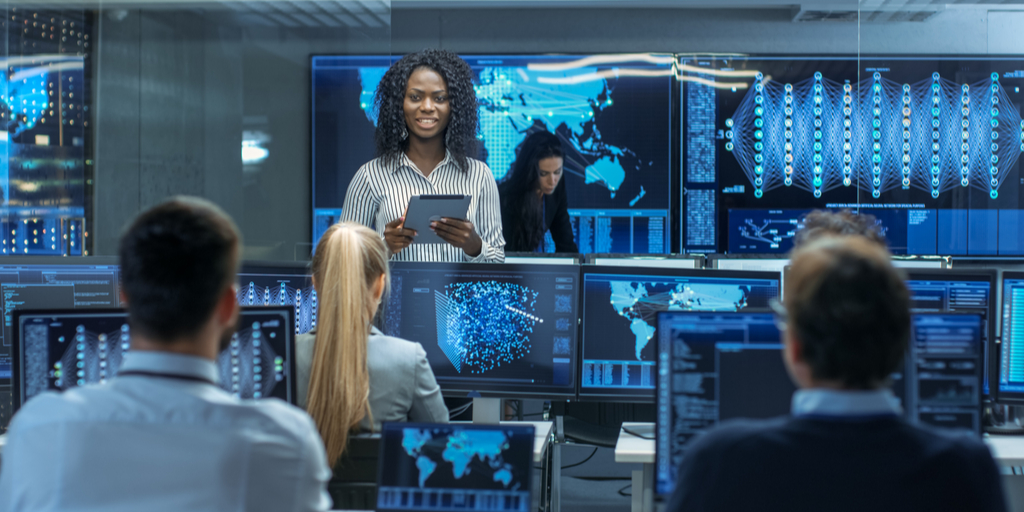
In the pulsating ecosystem of technological innovation, machine learning science emerges as a revolutionary discipline that promises to redefine the boundaries of human knowledge and technological potential. As artificial intelligence transforms from a futuristic concept to an omnipresent technological force, machine learning scientists stand at the epicenter of a profound technological revolution, architecting intelligent systems that can learn, adapt, and solve complex problems with unprecedented sophistication. This comprehensive exploration unveils the intricate world of machine learning science, offering aspiring professionals a strategic roadmap to navigate the complex, dynamic landscape of technological innovation in 2025 and beyond.
Understanding Machine Learning Science
Machine learning science represents a cutting-edge technological domain that transcends traditional computational boundaries, emerging as a sophisticated interdisciplinary field that synthesizes advanced mathematics, computer science, cognitive psychology, and statistical modeling. Unlike conventional programming paradigms, machine learning science focuses on creating intelligent systems capable of autonomous learning, pattern recognition, and predictive analysis without explicit programming instructions.
Professionals in this field are essentially cognitive architects, responsible for designing, developing, and implementing intelligent algorithms that can process vast amounts of data, identify complex patterns, and generate predictive insights across diverse technological ecosystems. The technological evolution of machine learning science has dramatically expanded beyond traditional computational limitations, positioning itself as a critical intersection between advanced algorithmic design, data analysis, and artificial intelligence technologies.
Modern machine learning scientists must navigate an increasingly complex technological landscape that demands expertise in advanced mathematical modeling, deep learning architectures, neural network design, probabilistic reasoning, and sophisticated data processing techniques. They are tasked with developing intelligent systems that can not only analyze and interpret massive datasets but also generate innovative solutions, drive strategic decision-making, and create transformative technological capabilities across multiple domains, from healthcare and finance to autonomous systems and predictive analytics.
Key Concepts to Learn
The foundational landscape of machine learning science demands a comprehensive understanding of complex technological frameworks and sophisticated methodological approaches that define this revolutionary discipline. The rapidly evolving ecosystem of machine learning requires professionals to develop a multifaceted skill set that integrates advanced mathematical knowledge, computational techniques, and innovative problem-solving strategies.
Advanced Mathematical Foundations: Develop deep understanding of linear algebra, calculus, probability theory, and statistical modeling techniques essential for sophisticated machine learning algorithm design.
Neural Network Architectures: Gain expertise in complex neural network designs, including deep learning frameworks, convolutional and recurrent neural networks, and generative adversarial network technologies.
Algorithmic Design: Master advanced algorithmic strategies, including supervised and unsupervised learning methodologies, reinforcement learning techniques, and probabilistic reasoning frameworks.
Data Processing and Feature Engineering: Learn sophisticated techniques for data preprocessing, feature selection, dimensionality reduction, and advanced feature engineering strategies.
Ethical AI and Responsible Machine Learning: Develop comprehensive understanding of algorithmic bias, ethical considerations, and responsible implementation of machine learning technologies across diverse technological ecosystems.
Educational Pathway
The journey to becoming a machine learning scientist in 2025 requires a revolutionary approach to education that transcends traditional academic boundaries, integrating advanced technological training, interdisciplinary knowledge acquisition, and continuous skill development. Successful professionals must create a comprehensive learning strategy that bridges theoretical understanding with practical implementation capabilities.
Recommended educational approaches include:
Specialized Academic Programs: Pursue advanced interdisciplinary degrees combining computer science, mathematics, cognitive science, and specialized machine learning curricula that offer comprehensive theoretical and practical training.
Professional Certifications: Obtain advanced certifications from leading technology platforms, including TensorFlow certification, AWS Machine Learning Specialty, and Google Professional Machine Learning Engineer credentials.
Online Learning Ecosystems: Leverage sophisticated online learning platforms like Coursera, edX, and specialized machine learning education providers offering comprehensive, industry-aligned curricula designed by global technological experts.
Research-Oriented Training: Engage in advanced research programs, academic collaborations, and specialized research initiatives that provide hands-on experience with cutting-edge machine learning technologies.
Continuous Learning Infrastructure: Develop a proactive approach to professional development through international conferences, specialized workshops, and emerging technology exploration platforms.
Practical Experience
Gaining practical experience represents a critical transformation point in establishing expertise within the complex field of machine learning science. Aspiring professionals must develop strategic approaches to translating theoretical knowledge into tangible technological innovations, creating comprehensive portfolios that demonstrate sophisticated problem-solving capabilities.
Personal Research Projects: Develop innovative machine learning projects addressing real-world challenges, utilizing advanced algorithmic techniques and publicly available datasets.
Kloudhire Platform: Leverage innovative platforms like Kloudhire, which connect emerging machine learning scientists with dynamic project opportunities, enabling practical skill development and professional networking across global technological ecosystems.
Open-Source Contributions: Actively participate in cutting-edge open-source machine learning projects, contributing to community-driven technological innovations and expanding professional networks.
Research Collaborations: Seek opportunities with academic institutions, technology companies, and research organizations focusing on advanced machine learning and artificial intelligence initiatives.
Competitive Programming Platforms: Engage with specialized machine learning competitions and challenge platforms that provide practical problem-solving experiences and industry recognition.
Skills to Develop
Success in machine learning science demands a sophisticated fusion of advanced technical expertise, mathematical precision, and innovative problem-solving capabilities. Professionals must cultivate a comprehensive skill set that transcends traditional computational boundaries and embraces the complex, interdisciplinary nature of intelligent technological systems.
Advanced Programming Proficiency: Master programming languages like Python, R, and Julia, with sophisticated expertise in machine learning libraries and frameworks.
Mathematical Modeling: Develop advanced mathematical modeling skills, including complex statistical analysis, probabilistic reasoning, and advanced optimization techniques.
Deep Learning Architectures: Gain comprehensive understanding of neural network design, including advanced architectures like transformers, generative models, and reinforcement learning systems.
Cloud and Distributed Computing: Develop expertise in cloud platforms and distributed computing technologies optimized for machine learning model training and deployment.
Interdisciplinary Communication: Cultivate skills to effectively communicate complex machine learning concepts across diverse professional and technical environments.
Job Opportunities in Machine Learning Science
The job market for machine learning science is experiencing significant growth and offers numerous opportunities for professionals. The demand for AI and machine learning specialists is skyrocketing, with job openings in these fields growing by 32% over the last five years. This high demand is driven by the transformative impact of AI across various industries, from space exploration to healthcare.
AI Research Scientist: Develop groundbreaking machine learning algorithms and contribute to fundamental technological innovations.
Applied Machine Learning Engineer: Design and implement practical machine learning solutions across healthcare, finance, robotics, and other critical domains.
Machine Learning Infrastructure Architect: Create sophisticated technological ecosystems that support advanced machine learning model development and deployment.
Ethical AI Specialist: Design and implement responsible machine learning strategies that address algorithmic bias and ethical considerations.
Cross-Domain Machine Learning Consultant: Provide strategic guidance on implementing machine learning technologies across diverse organizational contexts.
Conclusion
The path to becoming a machine learning scientist in 2025 represents a transformative journey of continuous learning, technological innovation, and intellectual curiosity. By developing a strategic approach to education, gaining practical experience, and cultivating a diverse, sophisticated skill set, aspiring professionals can position themselves at the forefront of technological revolution.
As intelligent technologies continue to reshape global technological landscapes, machine learning scientists will play an increasingly critical role in driving technological innovation, solving complex global challenges, and unlocking unprecedented possibilities of artificial intelligence. The fusion of advanced technical expertise, mathematical precision, and innovative problem-solving will differentiate successful professionals in this exciting and rapidly expanding field. Embrace the challenge, remain committed to continuous learning, and prepare to make significant contributions to the future of intelligent technological systems.